How to Create a Model Like LLaMA 2, LLaMA 3.1, or DeepSeek-R1
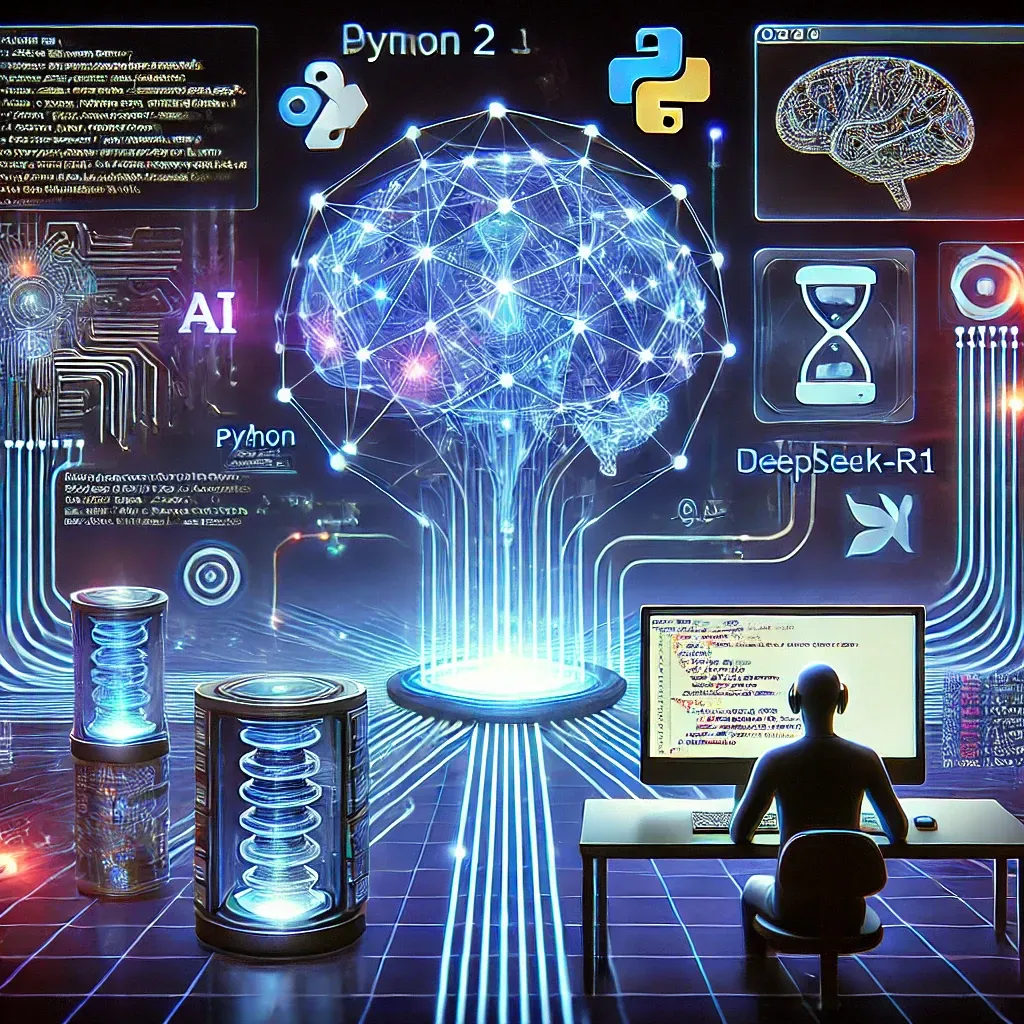
Introduction
Building a large-scale AI model like LLaMA 2, LLaMA 3.1, or DeepSeek-R1 requires expertise in machine learning, data processing, and optimization. This guide provides an in-depth look at training and fine-tuning AI models, covering data preparation, model architecture, and training using Python, as well as integration with PHP and JavaScript.
1. Understanding AI Model Training
Large language models (LLMs) are trained using:
✅ Massive datasets (text corpora, web pages, books).
✅ Transformer-based architectures like GPT, BERT, and LLaMA.
✅ High-performance hardware (GPUs/TPUs for acceleration).
✅ Fine-tuning for task-specific applications (chatbots, summarization, etc.).
The process consists of data preprocessing, model training, fine-tuning, and deployment.
2. Setting Up the Training Environment
To train a model from scratch, install key dependencies:
pip install torch transformers datasets accelerate tokenizers
Ensure you have access to a GPU (NVIDIA CUDA-enabled for performance).
3. Collecting and Preprocessing Data
AI models require clean and structured training data. Use publicly available datasets or custom text sources.
Python: Preprocessing Text Data
from datasets import load_dataset
dataset = load_dataset("wikipedia", "20220301.en")
# Convert text to lowercase and remove special characters
def preprocess_text(example):
example["text"] = example["text"].lower().replace("\n", " ")
return example
dataset = dataset.map(preprocess_text)
4. Defining the Model Architecture
We use Hugging Face’s Transformers library to define a model.
Python: Building a Transformer Model
from transformers import AutoModelForCausalLM, AutoTokenizer
model_name = "facebook/opt-1.3b" # LLaMA-like architecture
model = AutoModelForCausalLM.from_pretrained(model_name)
tokenizer = AutoTokenizer.from_pretrained(model_name)
5. Training the Model
Train the model using gradient accumulation and mixed precision training.
Python: Training a LLaMA-like Model
from transformers import Trainer, TrainingArguments
training_args = TrainingArguments(
output_dir="./llama_model",
evaluation_strategy="epoch",
per_device_train_batch_size=2,
save_steps=1000,
learning_rate=2e-5,
num_train_epochs=3,
fp16=True # Use mixed precision for speed
)
trainer = Trainer(
model=model,
args=training_args,
train_dataset=dataset["train"],
)
trainer.train()
6. Fine-Tuning for Custom Use Cases
After training, fine-tune on task-specific data (e.g., chatbots, summarization).
Python: Fine-Tuning Example
from transformers import TrainingArguments
finetune_args = TrainingArguments(
output_dir="./fine_tuned_model",
per_device_train_batch_size=4,
num_train_epochs=2,
save_steps=500,
)
trainer.args = finetune_args
trainer.train()
7. Deploying the Model Using API (PHP & JavaScript)
PHP: Calling the Trained Model via API
<?php
$url = 'http://localhost:8000/generate';
$data = json_encode(["prompt" => "Tell me about AI models"]);
$options = [
'http' => [
'header' => "Content-type: application/json\r\n",
'method' => 'POST',
'content' => $data
]
];
$context = stream_context_create($options);
$result = file_get_contents($url, false, $context);
echo $result;
?>
JavaScript: Fetching Model Response via API
async function queryModel(prompt) {
const response = await fetch("http://localhost:8000/generate", {
method: "POST",
headers: { "Content-Type": "application/json" },
body: JSON.stringify({ prompt: prompt })
});
const data = await response.json();
console.log("AI Response:", data.response);
}
queryModel("Explain fine-tuning in AI");
8. Optimizing for Performance & Scaling
🚀 Use quantization (4-bit/8-bit models) for lower memory usage.
🚀 Leverage distributed training across multiple GPUs.
🚀 Optimize inference with ONNX Runtime, TensorRT, or DeepSpeed.
Conclusion
Creating AI models like LLaMA 2, LLaMA 3.1, or DeepSeek-R1 requires:
✅ High-quality data preprocessing
✅ Optimized model architecture
✅ Efficient training and fine-tuning
✅ Seamless deployment via APIs
With the right tools and techniques, you can train custom AI models for chatbots, NLP, or any machine learning application.
🚀 Want help training AI models? Contact AKADATA for expert solutions!